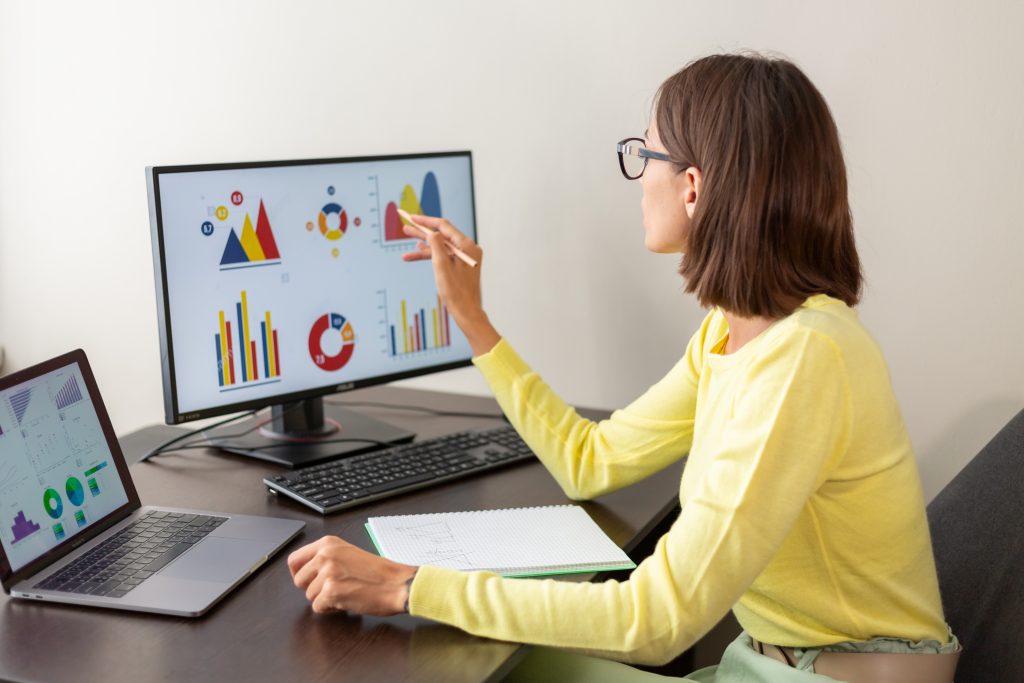
Predictive Analytics, as a concept, have been doing the rounds in the industry for decades. However, only recently has the power of Predictive Analytics been unleashed, and organisations are rapidly adopting the technology to give them a competitive advantage and increase bottom lines.
What is Predictive Analytics?
Simplistically put, Predictive Analytics is the process of extracting insights from data to discover complex associations, identify unseen patterns, forecast trends, identify associations, etc. It is the process of using data, statistics, powerful algorithms and machine learning techniques to identify future outcomes based on historical data. Powerful, accurate insights help organisations mitigate risks, identify areas of concern, and improve efficiencies.
Why is predictive analytics important?
Predictive analytics can help all types of organisations to help solve complex problems and uncover new opportunities. Common uses include:
Fraud Detection: Predictive Analytics can improve pattern detection and prevent criminal behaviour. With cybersecurity becoming a growing concern, organisations can benefit immensely as high-performance analytics examine all actions on a network in real-time to spot abnormalities that may indicate fraud, zero-day vulnerabilities, and advanced persistent threats.
Optimised Marketing Campaigns: Predictive analytics are used to identify trends in customer responses and purchases, which can reveal upselling opportunities. Predictive algorithms help businesses attract, retain and grow their most profitable customers.
Improved operations: Companies leverage predictive models to forecast inventory and manage resources. Predictive analytics have varied uses across industries; from setting ticket prices for airlines to predicting numbers of guests for any given night in the hotel industry. Predictive analytics is also used to predict machine lifecycle and faults to schedule preventive maintenance in industries. Predictive analytics enables organisations to function more efficiently.
Reduced risk: A well known example of the use of predictive analytics is credit scores which are used by companies to assess a buyer’s likelihood of default. Other risk-related uses are insurance claims and collections. Aside from the Financial Sector, Predictive Analytics is also used by companies to mitigate risks of equipment downtime, inventory shortage, etc.
Every predictive analytics algorithm results in predictions, but it is up to the developers and analysts to make these understandable and actionable. For organisations to make the most of this, it’s important to present actionable insights and exceptions in understandable formats, rather than flooding your customer with raw data.